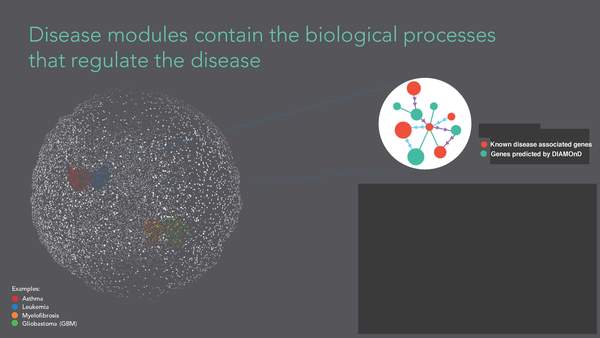
S. D. Ghiassian, J. Menche, A.-L. Barabási
A DIseAse MOdule Detection (DIAMOnD) Algorithm Derived from a Systematic Analysis of Connectivity Patterns of Disease Proteins in the Human Interactome
PLOS Computational Biology pcbi.1004120, 1-21 (2015)
Read the abstract
The observation that disease associated proteins often interact with each other has fueled the development of network-based approaches to elucidate the molecular mechanisms of human disease. Such approaches build on the assumption that protein interaction networks can be viewed as maps in which diseases can be identified with localized perturbation within a certain neighborhood. The identification of these neighborhoods, or disease modules, is therefore a prerequisite of a detailed investigation of a particular pathophenotype. While numerous heuristic methods exist that successfully pinpoint disease associated modules, the basic underlying connectivity patterns remain largely unexplored. In this work we aim to fill this gap by analyzing the network properties of a comprehensive corpus of 70 complex diseases. We find that disease associated proteins do not reside within locally dense communities and instead identify connectivity significance as the most predictive quantity. This quantity inspires the design of a novel Disease Module Detection (DIAMOnD) algorithm to identify the full disease module around a set of known disease proteins. We study the performance of the algorithm using well-controlled synthetic data and systematically validate the identified neighborhoods for a large corpus of diseases.
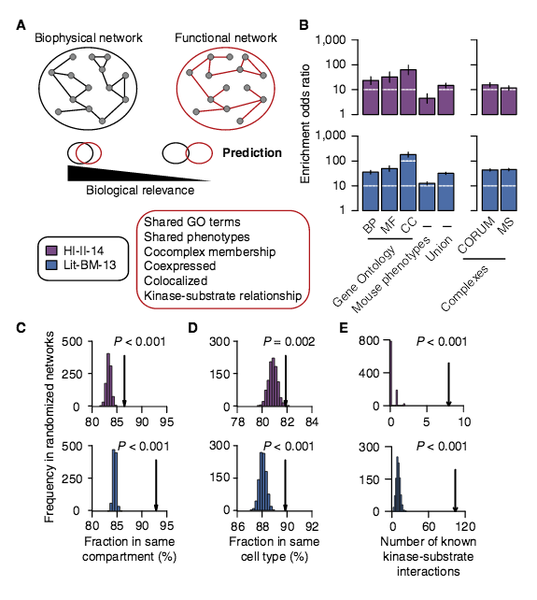
T. Rolland, M. Tasan, , B. Charloteaux, S. J. Pevzner,, Q. Zhong, N. Sahni, S. Yi,, I. Lemmens, C. Fontanillo,, R. Mosca, A. Kamburov, , S. D. Ghiassian, X. Yang,, L. Ghamsari, D. Balcha,, B. E. Begg, P. Braun, M. Brehm, M. P. Froly, A.-R. Carvunis, D, Convery-Zupan, R. Carominas,, J. Coulombe-Huntington, , E. Dann, M. Dreze, A. Dricot,, C. Fan, E. Franzosa, F. Gebrea, B. J. Gutierrez, M. F. Hardy,, M. Jin, S. Kang, R. Kiros, G. , Lin, K. Luck, A. MacWilliams,, J. Menche, R R. Murray, A., Palagi, M. M. Poulin, X. , Rambout, J. Rasla, P. Reichert, V. Romero, E. Ruyssinck, J. M., Sahalie, plus 20 more co-autho
A proteome-scale map of the human interactome network
Cell 159:5, 1212-1226 (2014)
Read the abstract
Just as reference genome sequences revolutionized human genetics, reference maps of interactome networks will be critical to fully understand genotype-phenotype relationships. Here, we describe a systematic map of ∼14,000 high-quality human binary protein-protein interactions. At equal quality, this map is ∼30% larger than what is available from small-scale studies published in the literature in the last few decades. While currently available information is highly biased and only covers a relatively small portion of the proteome, our systematic map appears strikingly more homogeneous, revealing a “broader” human interactome network than currently appreciated. The map also uncovers significant interconnectivity between known and candidate cancer gene products, providing unbiased evidence for an expanded functional cancer landscape, while demonstrating how high-quality interactome models will help “connect the dots” of the genomic revolution.
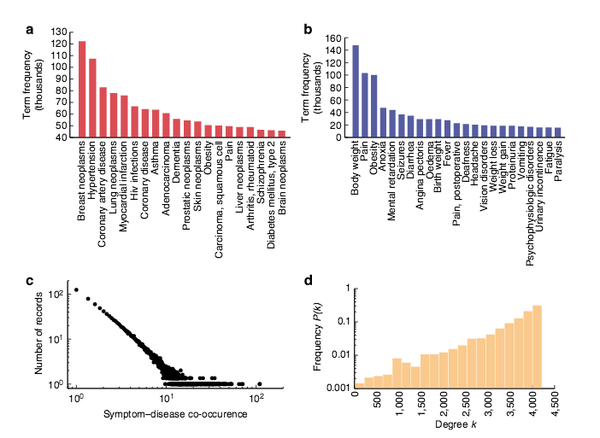
X. Z. Zhou, J. Menche, A.-L. Barabási, A. Sharma
Human symptoms–disease network
Nature Communications 5:4212, 1-10 (2014)
Read the abstract
In the post-genomic era, the elucidation of the relationship between the molecular origins of diseases and their resulting phenotypes is a crucial task for medical research. Here, we use a large-scale biomedical literature database to construct a symptom-based human disease network and investigate the connection between clinical manifestations of diseases and their underlying molecular interactions. We find that the symptom-based similarity of two diseases correlates strongly with the number of shared genetic associations and the extent to which their associated proteins interact. Moreover, the diversity of the clinical manifestations of a disease can be related to the connectivity patterns of the underlying protein interaction network. The comprehensive, high-quality map of disease–symptom relations can further be used as a resource helping to address important questions in the field of systems medicine, for example, the identification of unexpected associations between diseases, disease etiology research or drug design.
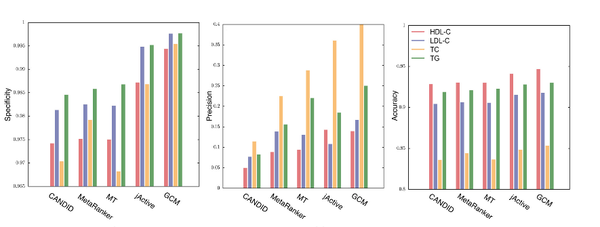
A. Sharma, N. Gulbahce, S. J. Pevzner, J. Menche, C. Ladenvall, L. Folkdersen, P. Eriksson, M. Orho-Melander, A.-L. Barabási
Network-based analysis of genome wide association data provides novel candidate genes for lipid and lipoprotein traits
Molecular & Cellular Proteomics 12, 3398-3408 (2013)
Read the abstract
Genome wide association studies (GWAS) identify susceptibility loci for complex traits, but do not identify particular genes of interest. Integration of functional and network information may help in overcoming this limitation and identifying new susceptibility loci. Using GWAS and comorbidity data, we present a network-based approach to predict candidate genes for lipid and lipoprotein traits. We apply a prediction pipeline incorporating interactome, co-expression, and comorbidity data to Global Lipids Genetics Consortium (GLGC) GWAS for four traits of interest, identifying phenotypically coherent modules. These modules provide insights regarding gene involvement in complex phenotypes with multiple susceptibility alleles and low effect sizes. To experimentally test our predictions, we selected four candidate genes and genotyped representative SNPs in the Malmö Diet and Cancer Cardiovascular Cohort. We found significant associations with LDL-C and total-cholesterol levels for a synonymous SNP (rs234706) in the cystathionine beta-synthase (CBS) gene (p = 1 × 10−5 and adjusted-p = 0.013, respectively). Further, liver samples taken from 206 patients revealed that patients with the minor allele of rs234706 had significant dysregulation of CBS (p = 0.04). Despite the known biological role of CBS in lipid metabolism, SNPs within the locus have not yet been identified in GWAS of lipoprotein traits. Thus, the GWAS-based Comorbidity Module (GCM) approach identifies candidate genes missed by GWAS studies, serving as a broadly applicable tool for the investigation of other complex disease phenotypes.
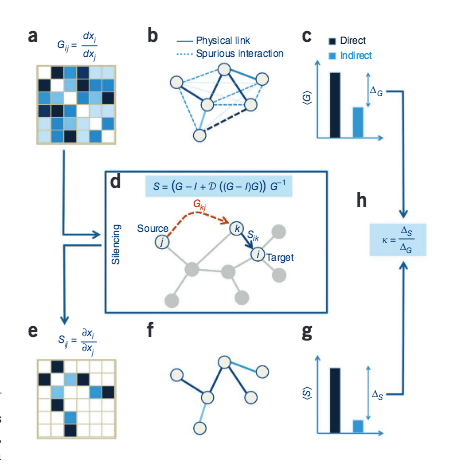
B. Barzel, A.-L. Barabási
Network link prediction by global silencing of indirect correlations
Nature Biotechnology 31: Num 8, 1-8 (2013)
Read the abstract
Predictions of physical and functional links between cellular components are often based on correlations between experimental measurements, such as gene expression. However, correlations are affected by both direct and indirect paths, confounding our ability to identify true pairwise interactions. Here we exploit the fundamental properties of dynamical correlations in networks to develop a method to silence indirect effects. The method receives as input the observed correlations between node pairs and uses a matrix transformation to turn the correlation matrix into a highly discriminative silenced matrix, which enhances only the terms associated with direct causal links. Against empirical data for Escherichia coli regulatory interactions, the method enhanced the discriminative power of the correlations by twofold, yielding >50% predictive improvement over traditional correlation measures and 6% over mutual information. Overall this silencing method will help translate the abundant correlation data into insights about a system's interactions, with applications ranging from link prediction to inferring the dynamical mechanisms governing biological networks.
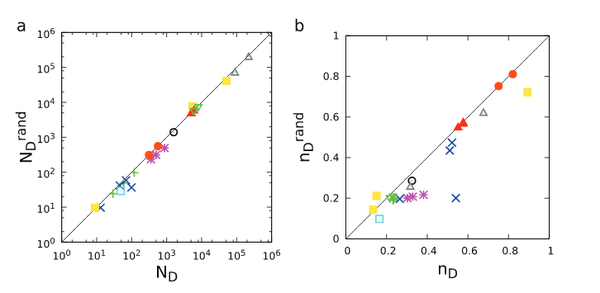
M. Pósfai, Y.-Y. Liu, J.-J. Slotine, A.-L. Barabási
Effect of correlations on network controllability
Scientific Reports 3:1067, 1-7 (2013)
Read the abstract
A dynamical system is controllable if by imposing appropriate external signals on a subset of its nodes, it can be driven from any initial state to any desired state in finite time. Here we study the impact of various network characteristics on the minimal number of driver nodes required to control a network. We find that clustering and modularity have no discernible impact, but the symmetries of the underlying matching problem can produce linear, quadratic or no dependence on degree correlation coefficients, depending on the nature of the underlying correlations. The results are supported by numerical simulations and help narrow the observed gap between the predicted and the observed number of driver nodes in real networks.
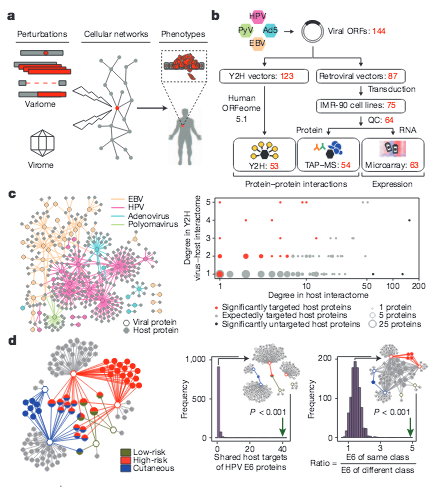
O. Rozenblatt-Rosen, R. C. Deo, M. Padi, G. Adelmant, T. Rolland, M. Grace, A. Dricot, M. Askenazi, M. Tavares, S. J. Pevzner, F. Abderazzaq, D. Byrdsong, A.-R. Carvunis, A. A. Chen, J. Cheng, M. Correll, M. Durate, C. Fan, M. C. Feltkamp, S. B. Ficarro, R. Franchi, B. K. Garg, N. Gulbahce, T. Hao, A. M. Holthaus, R. James, A. Korkhin, L. Litovchick, J. C. Mar, T. R. Pak, S. Rabello, R. Rubio, Y. Shen, S. Singh, J. M. Spangle, M. Tasan, S. Wanamakter, J. T. Webber, J. Roecklein-Canfield,, E. Johannsen, A.-L. Barabasi,, R. Beroukhim, E. Kieff,, M. E. Cusick, D. E. Hill,, K. Munger, J. A. Marto,, J. Quackenbush, F. P. Roth,, J. A. DeCaprio, M. Vidal
Interpreting cancer genomes using systematic host network perturbations by tumour virus proteins
Nature 487, 491-495 (2012)
Read the abstract
Genotypic differences greatly influence susceptibility and resistance to disease. Understanding genotype–phenotype relationships requires that phenotypes be viewed as manifestations of network properties, rather than simply as the result of individual genomic variations. Genome sequencing efforts have identified numerous germline mutations, and large numbers of somatic genomic alterations, associated with a predisposition to cancer. However, it remains difficult to distinguish background, or ‘passenger’, cancer mutations from causal, or ‘driver’, mutations in these data sets. Human viruses intrinsically depend on their host cell during the course of infection and can elicit pathological phenotypes similar to those arising from mutations. Here we test the hypothesis that genomic variations and tumour viruses may cause cancer through related mechanisms, by systematically examining host interactome and transcriptome network perturbations caused by DNA tumour virus proteins. The resulting integrated viral perturbation data reflects rewiring of the host cell networks, and highlights pathways, such as Notch signalling and apoptosis, that go awry in cancer. We show that systematic analyses of host targets of viral proteins can identify cancer genes with a success rate on a par with their identification through functional genomics and large-scale cataloguing of tumour mutations. Together, these complementary approaches increase the specificity of cancer gene identification. Combining systems-level studies of pathogen-encoded gene products with genomic approaches will facilitate the prioritization of cancer causing driver genes to advance the understanding of the genetic basis of human cancer.
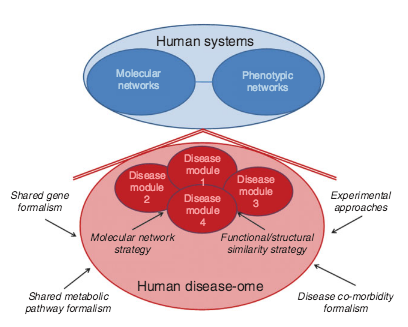
J. Loscalzo, A.-L. Barabási
Systems biology and the future of medicine
WIREs Systems Biology and Medicine 3, 619-627 (2011)
Read the abstract
Contemporary views of human disease are based on simple correlation between clinical syndromes and pathological analysis dating from the late 19th century. Although this approach to disease diagnosis, prognosis, and treatment has served the medical establishment and society well for many years, it has serious shortcomings for the modern era of the genomic medicine that stem from its reliance on reductionist principles of experimentation and analysis. Quantitative, holistic systems biology applied to human disease offers a unique approach for diagnosing established disease, defining disease predilection, and developing individualized (personalized) treatment strategies that can take full advantage of modern molecular pathobiology and the comprehensive data sets that are rapidly becoming available for populations and individuals. In this way, systems pathobiology offers the promise of redefining our approach to disease and the field of medicine.
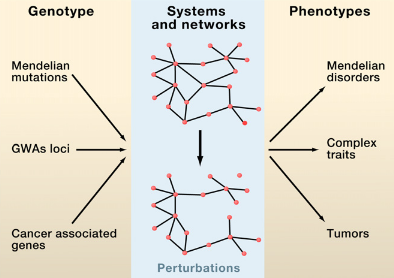
M. Vidal, M. E. Cusick, A.-L. Barabasi
Interactome Networks and Human Disease
Cell 144, 986-995 (2011)
Read the abstract
Complex biological systems and cellular networks may underlie most genotype to phenotype relationships. Here, we review basic concepts in network biology, discussing different types of interactome networks and the insights that can come from analyzing them. We elaborate on why interactome networks are important to consider in biology, how they can be mapped and integratedwith each other, what global properties are starting to emerge from interactome network models, and how these properties may relate to human disease.
J. J. Kahle, N. Gulbahce, C. A. Shaw, J. Lim, D. E. Hill, A.-L. Barabás, H. Y. Zoghbi
Comparison of an expanded ataxia interactome with patient medical records reveals a relationship between macular degeneration and ataxia
Human Molecular Genetics 20, 510-527 (2011)
Read the abstract
Spinocerebellar ataxias 6 and 7 (SCA6 and SCA7) are neurodegenerative disorders caused by expansion of CAG repeats encoding polyglutamine (polyQ) tracts in CACNA1A, the alpha1A subunit of the P/Q-type calcium channel, and ataxin-7 (ATXN7), a component of a chromatin-remodeling complex, respectively. We hypothesized that finding new protein partners for ATXN7 and CACNA1A would provide insight into the biology of their respective diseases and their relationship to other ataxia-causing proteins. We identified 118 protein interactions for CACNA1A and ATXN7 linking them to other ataxia-causing proteins and the ataxia network. To begin to understand the biological relevance of these protein interactions within the ataxia network, we used OMIM to identify diseases associated with the expanded ataxia network. We then used Medicare patient records to determine if any of these diseases co-occur with hereditary ataxia. We found that patients with ataxia are at 3.03-fold greater risk of these diseases than Medicare patients overall. One of the diseases comorbid with ataxia is macular degeneration (MD). The ataxia network is significantly (P= 7.37 × 10(-5)) enriched for proteins that interact with known MD-causing proteins, forming a MD subnetwork. We found that at least two of the proteins in the MD subnetwork have altered expression in the retina of Ataxin-7(266Q/+) mice suggesting an in vivo functional relationship with ATXN7. Together these data reveal novel protein interactions and suggest potential pathways that can contribute to the pathophysiology of ataxia, MD, and diseases comorbid with ataxia.
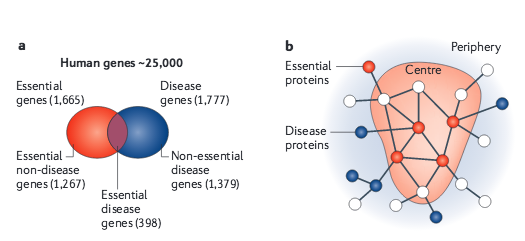
A.-L. Barabási, N. Gulbahce, J. Loscalzo
Network medicine: a network-based approach to human disease
Nature Reviews Genetics 12, 56-68 (2011)
Read the abstract
Given the functional interdependencies between the molecular components in a human cell, a disease is rarely a consequence of an abnormality in a single gene, but reflects the perturbations of the complex intracellular and intercellular network that links tissue and organ systems. The emerging tools of network medicine offer a platform to explore systematically not only the molecular complexity of a particular disease, leading to the identification of disease modules and pathways, but also the molecular relationships among apparently distinct (patho)phenotypes. Advances in this direction are essential for identifying new disease genes, for uncovering the biological significance of disease-associated mutations identified by genome-wide association studies and full-genome sequencing, and for identifying drug targets and biomarkers for complex diseases.
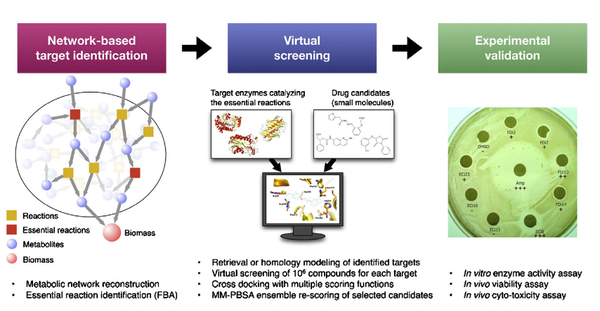
Y. Shen, L. Liu, G. Estiu, B. Isin, Y.-Y. Ahn, D.-S. Lee, A.-L. Barabásii, v. Kapatral, O. Wiest, Z. N. Oltvai
Blueprint for antimicrobial hit discovery targeting metabolic networks
Proceedings of the National Academy of Sciences of the United States of America 10.1073, 1-6 (2010)
Read the abstract
Advances in genome analysis, network biology, and computational chemistry have the potential to revolutionize drug discovery by combining system-level identification of drug targets with the atomistic modeling of small molecules capable of modulating their activity. To demonstrate the effectiveness of such a discovery pipeline, we deduced common antibiotic targets in Escherichia coli and Staphylococcus aureus by identifying shared tissue-specific or uniformly essential metabolic reactions in their metabolic networks. We then predicted through virtual screening dozens of potential inhibitors for several enzymes of these reactions and showed experimentally that a subset of these inhibited both enzyme activities in vitro and bacterial cell viability. This blueprint is applicable for any sequenced organism with high-quality metabolic reconstruction and suggests a general strategy for strain-specific antiinfective therapy.
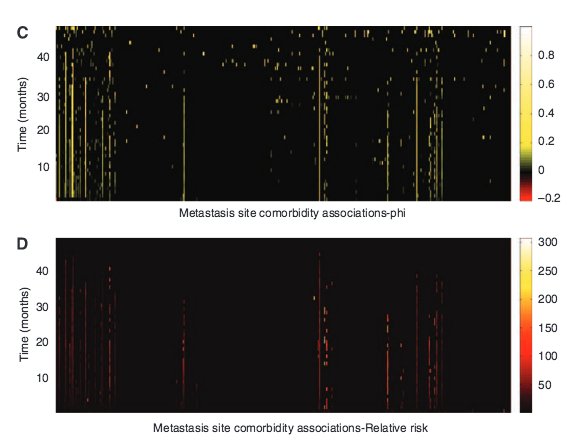
L. L. Chen, N. Blumm, N. A. Christakis, A.-L. Barabási, T. S. Deisboeck
Cancer metastasis networks and the prediction of progression patterns
British Journal of Cancer 101, 749-758 (2009)
Read the abstract
Background: Metastasis patterns in cancer vary both spatially and temporally. Network modeling may allow the incorporation of the temporal dimension in the analysis of these patterns.METHODS: We used Medicare claims of 2 265 167 elderly patients aged X65 years to study the large-scale clinical pattern of metastases. We introduce the concept of a cancer metastasis network, in which nodes represent the primary cancer site and the sites of subsequent metastases, connected by links that measure the strength of co-occurrence.RESULTS: These cancer metastasis networks capture both temporal and subtle relational information, the dynamics of which differ between cancer types. Using these networks as entities on which the metastatic disease of individual patients may evolve, we show that they may be used, for certain cancer types, to make retrograde predictions of a primary cancer type given a sequence ofmetastases, as well as anterograde predictions of future sites of metastasis.
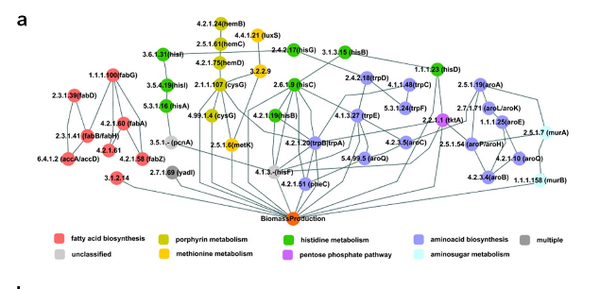
D.-S. Lee, H. Burd, J. Liu, E. Almass, O. Weist, A.-L. Barabási, Z. N. Oltvai, V. Kapatra
Comparative Genome-Scale Metabolic Reconstruction and Flux Balance Analysis of Multiple Staphylococcus aureus Genomes Identify Novel Antimicrobial Drug Targets
Journal of Bacteriology 191:12, 4015–4024 (2009)
Read the abstract
Mortality due to multidrug-resistant Staphylococcus aureus infection is predicted to surpass that of human immunodeficiency virus/AIDS in the United States. Despite the various treatment options for S. aureus infections, it remains a major hospital- and community-acquired opportunistic pathogen. With the emergence of multidrug-resistant S. aureus strains, there is an urgent need for the discovery of new antimicrobial drug targets in the organism. To this end, we reconstructed the metabolic networks of multidrug-resistant S. aureus strains using genome annotation, functional-pathway analysis, and comparative genomic approaches, followed by flux balance analysis-based in silico single and double gene deletion experiments. We identified 70 single enzymes and 54 pairs of enzymes whose corresponding metabolic reactions are predicted to be unconditionally essential for growth. Of these, 44 single enzymes and 10 enzyme pairs proved to be common to all 13 S. aureus strains, including many that had not been previously identified as being essential for growth by gene deletion experiments in S. aureus. We thus conclude that metabolic reconstruction and in silico analyses of multiple strains of the same bacterial species provide a novel approach for potential antibiotic target identification.
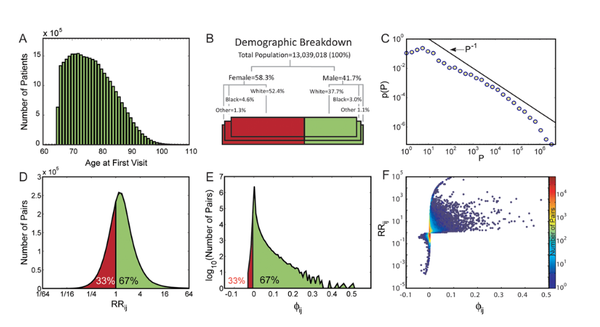
C. A. Hidalgo, N. Blumm, A.-L. Barabási, N. A. Christakis
A dynamic network approach for the study of human phenotypes
PLoS Computational Biology 5:4, 1-11 (2009)
Read the abstract
The use of networks to integrate different genetic, proteomic, and metabolic datasets has been proposed as a viable path toward elucidating the origins of specific diseases. Here we introduce a new phenotypic database summarizing correlations obtained from the disease history of more than 30 million patients in a Phenotypic Disease Network (PDN). We present evidence that the structure of the PDN is relevant to the understanding of illness progression by showing that (1) patients develop diseases close in the network to those they already have; (2) the progression of disease along the links of the network is different for patients of different genders and ethnicities; (3) patients diagnosed with diseases which are more highly connected in the PDN tend to die sooner than those affected by less connected diseases; and (4) diseases that tend to be preceded by others in the PDN tend to be more connected than diseases that precede other illnesses, and are associated with higher degrees of mortality. Our findings show that disease progression can be represented and studied using network methods, offering the potential to enhance our understanding of the origin and evolution of human diseases. The dataset introduced here, released concurrently with this publication, represents the largest relational phenotypic resource publicly available to the research community.
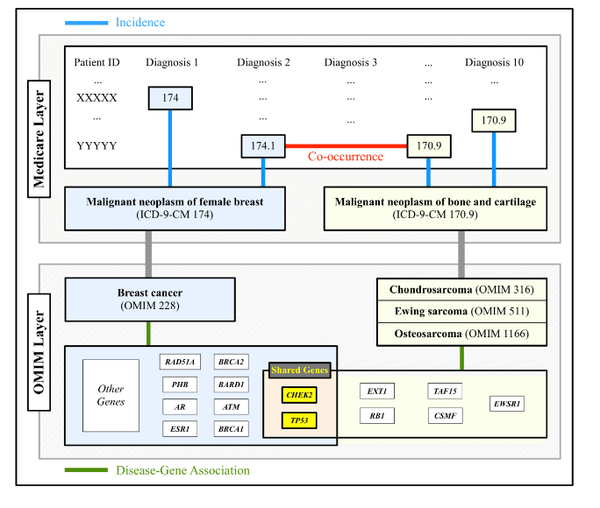
J. Park, D. S. Lee, N. A. Christakis, A.-L. Barabási
The impact of cellular networks on disease comorbidity
Molecular Systems Biology 5:262, 1-7 (2009)
Read the abstract
The impact of disease-causing defects is often not limited to the products of a mutated gene but, thanks to interactions between the molecular components, may also affect other cellular functions, resulting in potential comorbidity effects. By combining information on cellular interactions, disease--gene associations, and population-level disease patterns extracted from Medicare data, we find statistically significant correlations between the underlying structure of cellular networks and disease comorbidity patterns in the human population. Our results indicate that such a combination of population-level data and cellular network information could help build novel hypotheses about disease mechanisms.
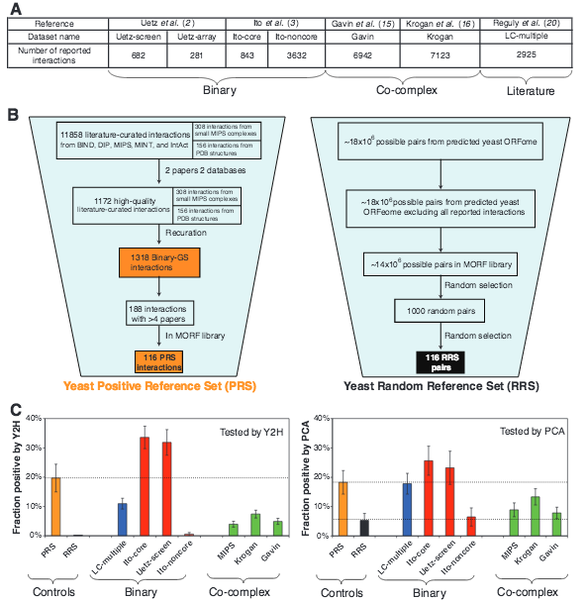
H. Yu, P. Braun, M. A. Yildirim, I. Lemmens, K. Venkatesan, J. Sahalie, T. Hirozane-Kishikawa, F. Gebreab, N. Li, N. Simonis, T. Hao, J.-F. Raul, A. Dricot, A. Vazquez, R. R. Murray, C. Simon, L. Tardivo, S. Tam, N. Svrzikapa, C. Fan, A.-S. de Semt, A. Motyl, M. E. Hudson, J. Park, X. Xin, M. E. Cusick, T. Moore, C. Boone, M. Snyder, F. P. Roth, A.-L. Barabási, J. Tavernier, D. E. Hill, M. Vidal
High-Quality Binary Protein Interaction Map of the Yeast Interactome Network
Science 322, 104-110 (2008)
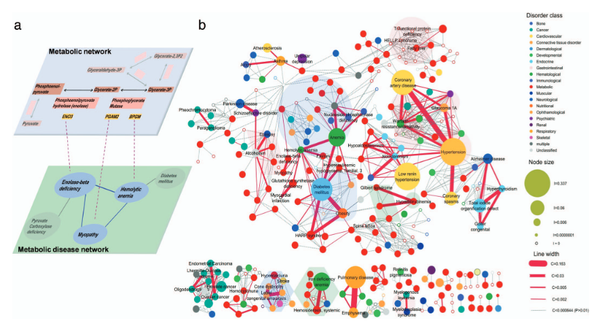
D.-S. Lee, J. Park, K. A. Kay, N. A. Christakis, Z. N. Oltvai, A.-L. Barabási
The implications of human metabolic network topology for disease comorbidity
Proceedings of the National Academy of Sciences 105, 9880-9885 (2008)
Read the abstract
Most diseases are the consequence of the breakdown of cellular processes, but the relationships among genetic/epigenetic defects, the molecular interaction networks underlying them, and the disease phenotypes remain poorly understood. To gain insights into such relationships, here we constructed a bipartite human disease association network in which nodes are diseases and two diseases are linked if mutated enzymes associated with them catalyze adjacent metabolic reactions. We find that connected disease pairs display higher correlated reaction flux rate, corresponding enzyme-encoding gene coexpression, and higher comorbidity than those that have no metabolic link between them. Furthermore, the more connected a disease is to other diseases, the higher is its prevalence and associated mortality rate. The network topology-based approach also helps to
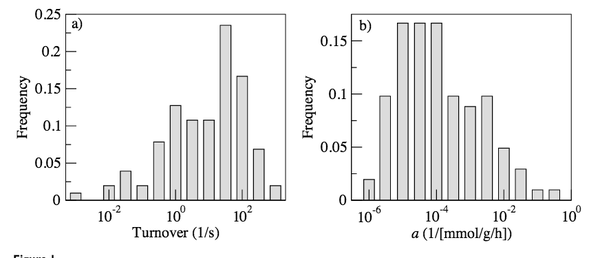
A. Vazquez, Q. K. Beg, M. A. de Menezes, J. Ernst, Z. Bar-Joseph, A.-L. Barabási, L. G. Boros, Z. N. Oltvai
Impact of the solvent capacity constraint on E. coli metabolism
BMC Systems Biology 2:7, 1-10 (2008)
Read the abstract
Obtaining quantitative predictions for cellular metabolic activities requires the identification and modeling of the physicochemical constraints that are relevant at physiological growth conditions. Molecular crowding in a cell's cytoplasm is one such potential constraint, as it limits the solvent capacity available to metabolic enzymes.
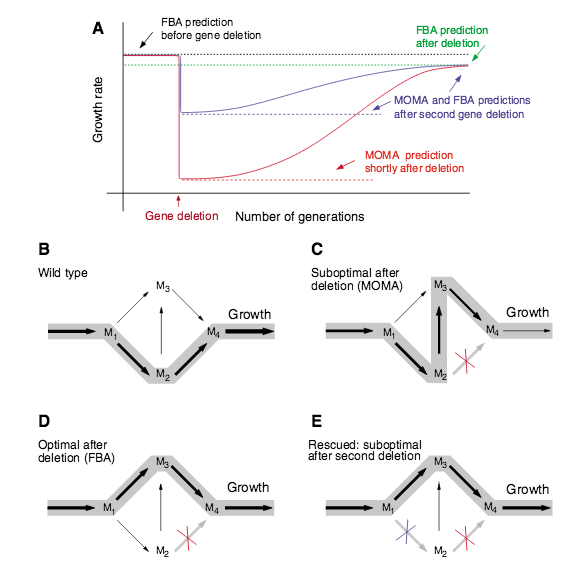
A. Motter, N. Gulbahce, E. Almaas, A.-L. Barabási
Predicting synthetic rescues in metabolic networks
Molecular Systems Biology 4:168, 1-10 (2008)
Read the abstract
An important goal of medical research is to develop methods to recover the loss of cellular function due to mutations and other defects. Many approaches based on gene therapy aim to repair the defective gene or to insert genes with compensatory function. Here, we propose an alternative, network-based strategy that aims to restore biological function by forcing the cell to either bypass the functions affected by the defective gene, or to compensate for the lost function. Focusing on the metabolism of single-cell organisms, we computationally study mutants that lack an essential enzyme, and thus are unable to grow or have a significantly reduced growth rate. We show that several of these mutants can be turned into viable organisms through additional gene deletions that restore their growth rate. In a rather counterintuitive fashion, this is achieved via additional damage to the metabolic network. Using flux balance-based approaches, we identify a number of synthetically viable gene pairs, in which the removal of one enzyme-encoding gene results in a non-viable phenotype, while the deletion of a second enzyme-encoding gene rescues the organism. The systematic network-based identification of compensatory rescue effects may open new avenues for genetic interventions.
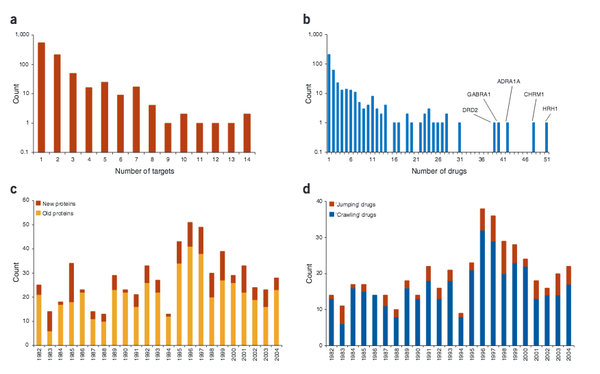
M. A. Yildirim, K.-L. Goh, M.E. Cusick, A.-L. Barabási, M. Vidal
Drug-target network
Nature Biotechnology 25:10, 1119-1126 (2007)
Read the abstract
The global set of relationships between protein targets of all drugs and all disease-gene products in the human protein–protein interaction or ‘interactome’ network remains uncharacterized. We built a bipartite graph composed of US Food and Drug Administration–approved drugs and proteins linked by drug–target binary associations. The resultingnetwork connects most drugs into a highly interlinked giant component, with strong local clustering of drugs of similar types according to Anatomical Therapeutic Chemical classification. Topological analyses of this network quantitatively showed an overabundance of ‘follow-on’ drugs, that is, drugs that target already targeted proteins. By including drugs currently under investigation, we identified a trend toward more functionally diverse targets improving polypharmacology. To analyze the relationships between drug targets and disease-gene products, we measured the shortest distance between both sets of proteins in current models of the human interactome network. Significant differences in distance were found between etiological and palliative drugs. A recent trend toward more rational drug design was observed.
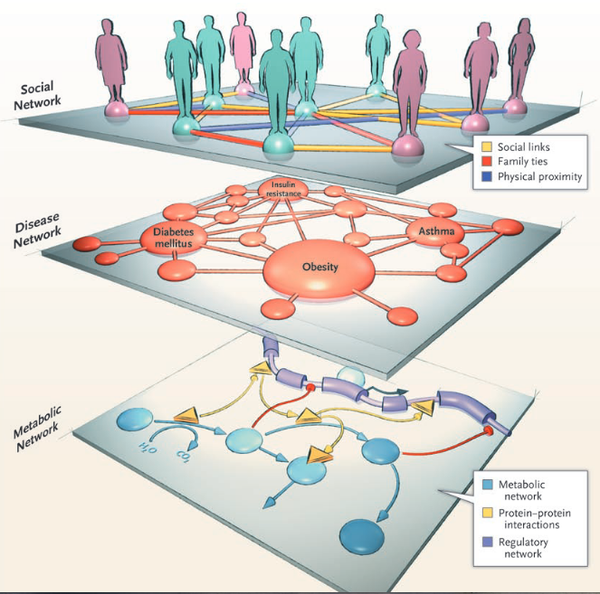
A.L. Barabási
Network Medicine — From Obesity to the “Diseasome”
New England Journal of Medicine 357, 404-407 (2007)
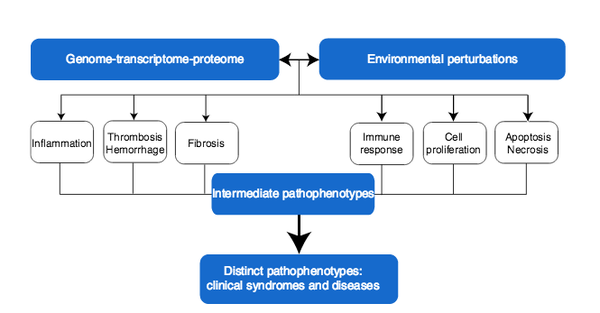
J. Loscalzo, I. Kohane, A.-L. Barabási
Human disease classification in the postgenomic era: A complex systems approach to human pathobiology
Molecular Systems Biology 3:124, 1-11 (2007)
Read the abstract
Contemporary classification of human disease derives from observational correlation between pathological analysis and clinical syndromes. Characterizing disease in this way established a nosology that has served clinicians well to the current time, and depends on observational skills and simple laboratory tools to define the syndromic phenotype. Yet, this time-honored diagnostic strategy has significant shortcomings that reflect both a lack of sensitivity in identifying preclinical disease, and a lack of specificity in defining disease unequivocally. In this paper, we focus on the latter limitation, viewing it as a reflection both of the different clinical presentations of many diseases (variable phenotypic expression), and of the excessive reliance on Cartesian reductionism in establishing diagnoses. The purpose of this perspective is to provide a logical basis for a new approach to classifying human disease that uses.
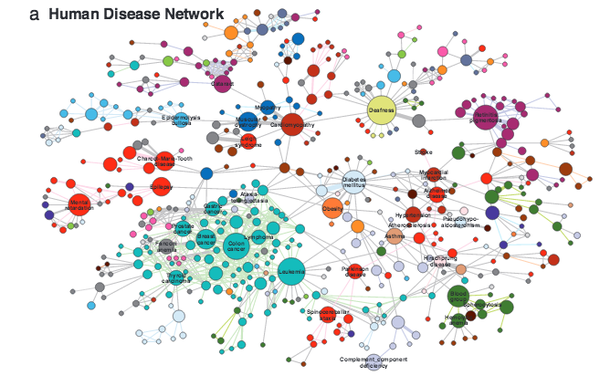
K.-I. Goh, M. E. Cusick, D. Valle, B. Childs, M. Vidal, A.-L. Barabási
The human disease network
Proceedings of the National Academy of Sciences 104:21, 8685 (2007)
Read the abstract
A network of disorders and disease genes linked by known disorder–gene associations offers a platform to explore in a single graphtheoretic framework all known phenotype and disease gene associations, indicating the common genetic origin of many diseases. Genes associated with similar disorders show both higher likelihood of physical interactions between their products and higher expression profiling similarity for their transcripts, supporting the existence of distinct disease-specific functional modules. We find that essential human genes are likely to encode hub proteins and are expressed widely in most tissues. This suggests that disease genes also would play a central role in the human interactome. In contrast, we find that the vast majority of disease genes are nonessential and show no tendency to encode hub proteins, and their expression pattern indicates that they are localized in the functional periphery of the network. A selection-based model explains the observed difference between essential and disease genes and also suggests that diseases caused by somatic mutations should not be peripheral, a prediction we confirm for cancer genes.
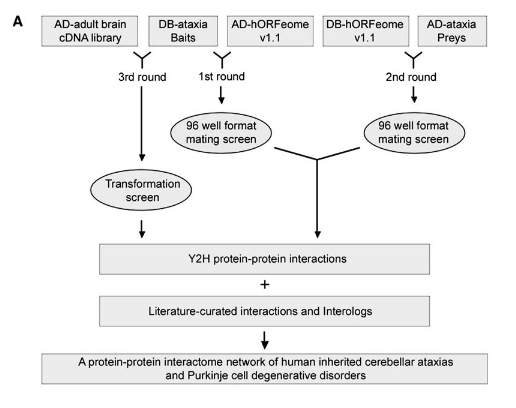
J. Lim, T. Hao, C. Shaw, A.J. Patel, G. Szabo, J.F. Rual, C.J. Fisk, N. Li, A. Smolyar, D.E. Hill, A.-L. Barabási, M. Vidal, H.Y. Zoghbi
A protein-protein interaction network for human inherited ataxias and disorders of Purkinje cell degeneration
Cell 125, 801-814 (2006)
Read the abstract
Many human inherited neurodegenerative disorders are characterized by loss of balance due to cerebellar Purkinje cell (PC) degeneration. Although the disease-causing mutations have been identified for a number of these disorders, the normal functions of the proteins involved remain, in many cases, unknown. To gain insight into the function of proteins involved in PC degeneration, we developed an interaction network for 54 proteins involved in 23 inherited ataxias and expanded the network by incorporating literature-curated and evolutionarily conserved interactions. We identified 770 mostly novel protein–protein interactions using a stringent yeast two-hybrid screen; of 75 pairs tested, 83% of the interactions were verified in mammalian cells. Many ataxia-causing proteins share interacting partners, a subset of which have been found to modify neurodegeneration in animal models. This interactome thus provides a tool for understanding pathogenic mechanisms common for this class of neurodegenerative disorders and for identifying candidate genes for inherited ataxias.